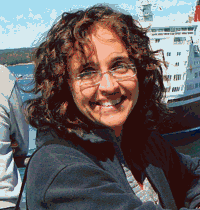
Quantum Reservoir Computing
Roberta Zambrini, University of the Balearic Islands
Reservoir computing is a supervised machine learning approach rooted in the framework of neural networks and demonstrated in several complex physical (analogue) reservoirs. This unconventional computing method allows for excellent performance in temporal processing series, as in speech recognition and forecasting, with an easy training strategy. Recent developments in reservoir computing and extreme learning machines are moving from classical to quantum implementations, being these also possible in NISQ devices.The first proposal of quantum reservoir computing was based on qubits, in a disordered transverse Ising model. It displayed a promising performance [1], even with few qubits, exploiting the Hilbert space dimension of quantum states. To achieve this performance, it was then found to be critical the ability of the system to thermalize, being many-body localization detrimental for the requirements of quantum reservoir computing [2]. For implementations of sequential (online) temporal series processing, a key issue is monitoring the output efficiently when including the effect of measurement. We will present strategies to overcome this challenge based on weak measurements [3].
[1] K. Fujii and K. Nakajima, Physical Review Applied 8, 024030 (2017).
[2] R. Martínez-Peña, et al. Physical Review Letters 127, 100502 (2021).
[3] P Mujal, R Martínez-Peña, GL Giorgi, MC Soriano, R Zambrini, arXiv:2205.06809, to be published in npj Quantum Information (2023)