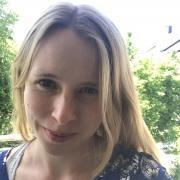
Insights from machine learning for quantum many body physics
Annabelle Bohrdt, ITAMP Harvard
Recent advances in quantum simulation experiments have paved the way for a new perspective on strongly correlated quantum many-body systems. Digital as well as analog quantum simulation platforms are capable of preparing desired quantum states, and various experiments are starting to explore equilibrium states as well as non-equilibrium many-body dynamics in previously inaccessible regimes. State-of-the art quantum simulators provide single-site resolved quantum projective measurements of the state, and thus a huge amount of information on the quantum many-body state. In this talk, I will introduce a novel, fully interpretable neural network architecture, the correlator convolutional neural network (CCNN), to analyze quantum snapshots. I will discuss a variety of applications ranging from the non-equilibrium dynamics in disordered systems to the doped Fermi-Hubbard model. I will then focus on the combination of the CCNN with confusion learning, which provides a tool to detect the possible existence and location of phase transitions and crossovers. In particular, I will show recent results on signatures of the pseudo- or spin-gap in quantum snapshots. The methods introduced here are very generally applicable and can potentially be useful to explore quantum data without potential bias, while taking into account all the information available.