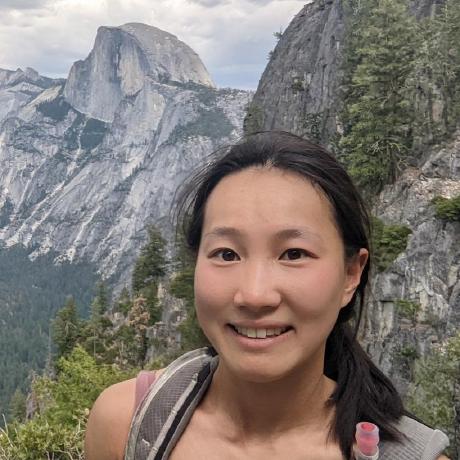
Learning topological states using variational tensor network tomography
Yanting Teng, Harvard University
Learning faithful representations of quantum states is crucial to fully characterizing the variety of many-body states created on quantum processors. Various tomographic methods face unique limitations in detecting topologically ordered two-dimensional states. In this talk, I investigate a heuristic tomographic method that combines variational optimization on tensor networks with randomized measurement techniques. I will discuss this approach’s capability of learning the ground state of the surface code Hamiltonian as well as an experimentally realizable quantum spin liquid state. I will show numerical results using MPS ansätze and systematically investigate the sample complexity required to achieve high fidelities for systems of sizes up to 48 qubits. In addition, we have theoretical insights into the scaling of our learning algorithm by analyzing the statistical properties of maximum likelihood estimation. Notably, our method is sample-efficient and experimentally friendly, only requiring snapshots of the quantum state measured randomly in the X or Z bases. I will provide intuitions for our proof that random-XZ measurements are tomographically complete for such states.